The integration of advanced technologies into drug discovery and development has led to the emergence of sophisticated tools that enhance the efficiency of pharmaceutical research. One such powerful tool is the LLM knowledg graph drug purpose, which bridges the gap between vast biological data and actionable insights. This article explores the role of LLM knowledg graph drug purpose in drug discovery, its applications, and how it is revolutionizing the pharmaceutical industry.
Table of Contents
Understanding the LLM Knowledge Graph Drug Purpose
In the field of pharmaceutical research, the LLM knowledge graph drug purpose refers to a specialized form of knowledge graph that is powered by large language models (LLMs) to map out relationships between different biological entities such as genes, proteins, diseases, and drugs. It utilizes natural language processing (NLP) and machine learning (ML) algorithms to analyze extensive datasets, creating a structured, interlinked graph of knowledge.
The LLM knowledge graph drug purpose serves multiple functions in drug research. By connecting various elements of biological data, it provides researchers with insights into the potential effects of drugs, their mechanisms of action, and their ability to treat specific diseases. The core advantage of a LLM knowledge graph drug purpose lies in its ability to extract meaningful patterns from complex, high-dimensional data, which can significantly speed up the drug discovery process.
How LLM Knowledge Graph Drug Purpose Enhances Drug Discovery
Drug discovery is a time-consuming and expensive process that typically involves extensive laboratory research, clinical trials, and a lot of guesswork. Traditional methods often rely on isolated pieces of information, making it challenging to understand the broader context of a drug’s potential impact. This is where the LLM knowledge graph drug purpose comes into play. By organizing diverse sources of information, the knowledge graph can provide researchers with a more holistic view of the drug discovery landscape.
For example, a LLM knowledge graph drug purpose can connect the genetic profiles of patients with data about how certain drugs interact with specific biomarkers. This enables researchers to identify new drug targets, predict drug efficacy, and even suggest potential drug repurposing opportunities. Through the analysis of structured and unstructured data, the LLM knowledge graph drug purpose aids in reducing the risk of late-stage drug development failures, thus saving both time and resources.
Key Components of LLM Knowledge Graph Drug Purpose
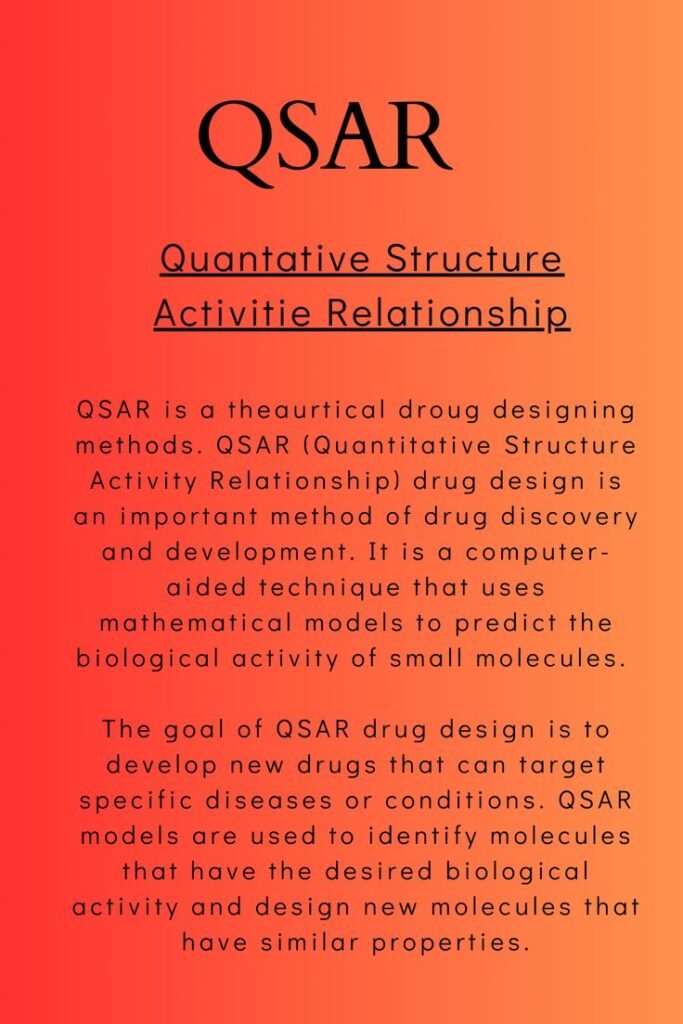
The LLM knowledge graph drug purpose is built on several key components that make it an invaluable tool for drug discovery:
- Entities and Nodes: The knowledge graph consists of entities such as drugs, diseases, proteins, genes, and biomarkers, each represented as nodes in the graph. These nodes are interconnected through edges, which represent relationships between them.
- Data Sources: The knowledge graph integrates diverse data sources, including scientific literature, clinical trial data, gene expression databases, and protein interaction networks. The LLM knowledge graph drug purpose uses natural language processing techniques to extract valuable information from both structured and unstructured datasets.
- Inference and Reasoning: The LLM knowledge graph drug purpose employs machine learning models to infer new relationships and potential drug repurposing opportunities. These models continuously evolve as more data is added, enhancing the accuracy of predictions over time.
- Visualization: The power of a knowledge graph lies in its ability to visualize complex relationships in an intuitive way. By presenting the data in an easily digestible format, researchers can quickly identify trends, connections, and gaps in the existing knowledge.
Applications of LLM Knowledge Graph Drug Purpose
The LLM knowledge graph drug purpose finds applications across various stages of drug discovery and development, contributing significantly to the acceleration and optimization of the entire process. Some of the notable applications include:
Drug Target Identification
The identification of novel drug targets is a critical first step in drug discovery. The LLM knowledge graph drug purpose can identify potential drug targets by analyzing patterns in genetic, proteomic, and clinical data. By mapping the relationships between genes, proteins, and diseases, it provides a comprehensive understanding of how different biological molecules interact. This aids in identifying new pathways or biomarkers that could be targeted by drugs.
Drug Repurposing
Drug repurposing, also known as drug repositioning, is the process of finding new uses for existing drugs. The LLM knowledge graph drug purpose can help researchers identify potential repurposing opportunities by uncovering hidden connections between drugs and diseases. For instance, a drug designed for one disease may have an unexpected effect on another disease. By analyzing the vast amount of biological and clinical data, the knowledge graph reveals these relationships and suggests new therapeutic indications for existing drugs.
Predicting Drug Efficacy and Safety
One of the major challenges in drug development is predicting the efficacy and safety of a drug before it enters clinical trials. The LLM knowledge graph drug purpose can be used to predict how a drug will behave in different biological contexts by analyzing data on similar drugs, their targets, and patient populations. By connecting data from preclinical studies, clinical trials, and real-world evidence, the knowledge graph provides insights into potential side effects, drug interactions, and the likelihood of success in treating specific diseases.
Personalized Medicine
Personalized medicine aims to tailor treatments to individual patients based on their genetic, environmental, and lifestyle factors. The LLM knowledge graph drug purpose plays a crucial role in this area by integrating patient-specific data with drug information. By mapping the genetic variations that influence drug responses, the knowledge graph helps researchers develop more effective and safer treatments for specific patient subgroups. This personalized approach reduces the trial-and-error process in drug selection, leading to better outcomes for patients.
Challenges and Limitations of LLM Knowledge Graph Drug Purpose
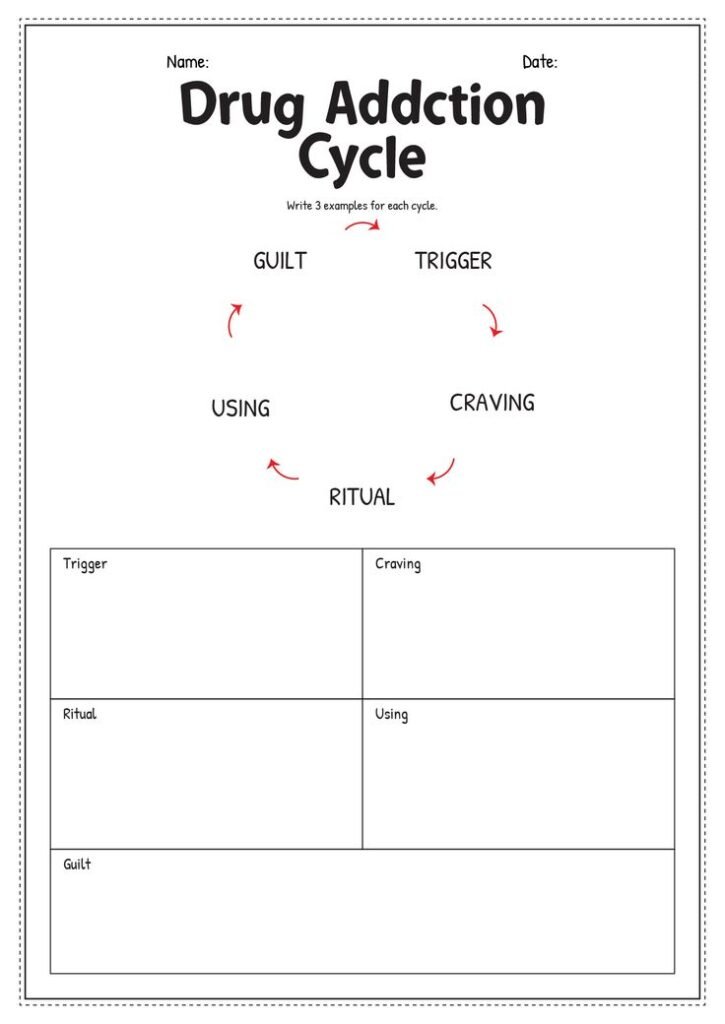
While the LLM knowledge graph drug purpose offers tremendous potential, it is not without challenges. Some of the key limitations include:
Data Quality and Completeness
The success of the LLM knowledge graph drug purpose is highly dependent on the quality and completeness of the data it is built on. Inaccurate, outdated, or incomplete data can lead to erroneous predictions and conclusions. Ensuring that the data used to build the knowledge graph is accurate and up-to-date is a continuous challenge for researchers.
Scalability
As the amount of biological data continues to grow, scaling the LLM knowledge graph drug purpose to handle large datasets becomes a significant challenge. Processing and storing massive volumes of data require advanced computational resources and sophisticated algorithms to maintain the speed and efficiency of the knowledge graph.
Interpretability
While the LLM knowledge graph drug purpose can uncover complex relationships within the data, the interpretability of these results remains a challenge. The predictions made by the graph may not always be easy to explain, making it difficult for researchers to trust the conclusions drawn. Enhancing the interpretability of the knowledge graph is an area of active research.
Future Prospects of LLM Knowledge Graph Drug Purpose
The potential of the LLM knowledge graph drug purpose in drug discovery and development is vast. As more data becomes available and machine learning algorithms continue to improve, the accuracy and utility of these knowledge graphs will only increase. With advancements in artificial intelligence and natural language processing, the integration of LLM knowledge graph drug purpose into pharmaceutical research is expected to revolutionize the industry.
In the near future, we can expect to see more personalized and efficient drug development processes. By combining the power of LLM knowledge graph drug purpose with other emerging technologies such as genomics, AI-driven drug design, and clinical trial optimization, the pharmaceutical industry will be better equipped to address unmet medical needs and create treatments tailored to individual patients.
Also read Did Trains Leave the Hurricane Path Earlu? Understanding Evacuations and Disruptions
Conclusion
The LLM knowledge graph drug purpose is a game-changer in the field of drug discovery and development. By leveraging the power of large language models and knowledge graphs, this tool enables researchers to unlock valuable insights from complex biological data. From drug target identification to personalized medicine, the LLM knowledge graph drug purpose has the potential to accelerate the development of new, more effective treatments while minimizing the risks associated with drug development. While there are still challenges to overcome, the future of the LLM knowledge graph drug purpose looks promising, with the potential to revolutionize the pharmaceutical industry and improve patient outcomes worldwide.